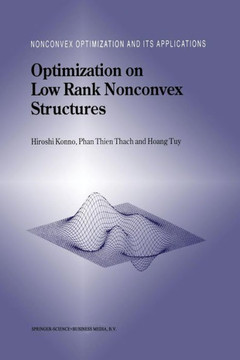
Springer
Robust Subspace Estimation Using Low-Rank Optimization: Theory and Applications
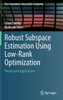
Robust Subspace Estimation Using Low-Rank Optimization: Theory and Applications
Various fundamental applications in computer vision and machine learning require finding the basis of a certain subspace. Examples of such applications include face detection, motion estimation, and activity recognition. An increasing interest has been recently placed on this area as a result of significant advances in the mathematics of matrix rank optimization. Interestingly, robust subspace estimation can be posed as a low-rank optimization problem, which can be solved efficiently using techniques such as the method of Augmented Lagrange Multiplier. In this book, the authors discuss fundamental formulations and extensions for low-rank optimization-based subspace estimation and representation. By minimizing the rank of the matrix containing observations drawn from images, the authors demonstrate how to solve four fundamental computer vision problems, including video denosing, background subtraction, motion estimation, and activity recognition.
Author: Omar Oreifej |
Publisher: Springer |
Publication Date: Apr 03, 2014 |
Number of Pages: 114 pages |
Binding: Hardback or Cased Book |
ISBN-10: 3319041835 |
ISBN-13: 9783319041834 |