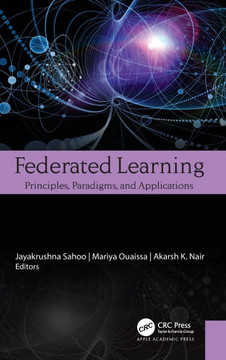
MDPI AG
Federated and Transfer Learning Applications
Product Code:
9783725800759
ISBN13:
9783725800759
Condition:
New
$86.66
$77.11
Sale 11%
The classic example of machine learning is based on isolated learning-a single model for each task using a single dataset. Most deep learning methods require a significant amount of labeled data, preventing their applicability in many areas where there is a shortage. In these cases, the ability of models to leverage information from unlabeled data or data that are not publicly available (for privacy and security reasons) can offer a remarkable alternative. Transfer learning and federated learning are alternative approaches that have emerged in recent years. More precisely, transfer learning is defined as the set of methods that leverage data from additional fields or tasks to train a model with greater generalizability and usually use a smaller amount of labeled data (via fine-tuning) to make them more specific for dedicated tasks. Accordingly, federated learning is a learning model that seeks to address the problem of data management and privacy through joint training with these data without the need to transfer the data to a central entity. With this in mind, this Special Issue of Applied Sciences provides an overview of the latest developments in this field.
Author: George Drosatos, Pavlos S Efraimidis, Avi Arampatzis |
Publisher: Mdpi AG |
Publication Date: Apr 01, 2024 |
Number of Pages: NA pages |
Language: English |
Binding: Hardcover |
ISBN-10: 3725800758 |
ISBN-13: 9783725800759 |
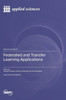
Federated and Transfer Learning Applications
$86.66
$77.11
Sale 11%
The classic example of machine learning is based on isolated learning-a single model for each task using a single dataset. Most deep learning methods require a significant amount of labeled data, preventing their applicability in many areas where there is a shortage. In these cases, the ability of models to leverage information from unlabeled data or data that are not publicly available (for privacy and security reasons) can offer a remarkable alternative. Transfer learning and federated learning are alternative approaches that have emerged in recent years. More precisely, transfer learning is defined as the set of methods that leverage data from additional fields or tasks to train a model with greater generalizability and usually use a smaller amount of labeled data (via fine-tuning) to make them more specific for dedicated tasks. Accordingly, federated learning is a learning model that seeks to address the problem of data management and privacy through joint training with these data without the need to transfer the data to a central entity. With this in mind, this Special Issue of Applied Sciences provides an overview of the latest developments in this field.
Author: George Drosatos, Pavlos S Efraimidis, Avi Arampatzis |
Publisher: Mdpi AG |
Publication Date: Apr 01, 2024 |
Number of Pages: NA pages |
Language: English |
Binding: Hardcover |
ISBN-10: 3725800758 |
ISBN-13: 9783725800759 |