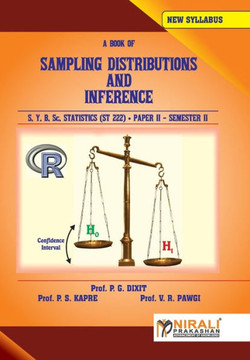
Springer
Statistical Inference Based on Kernel Distribution Function Estimators
Product Code:
9789819918614
ISBN13:
9789819918614
Condition:
New
$60.77
This book presents a study of statistical inferences based on the kernel-type estimators of distribution functions. The inferences involve matters such as quantile estimation, nonparametric tests, and mean residual life expectation, to name just some. Convergence rates for the kernel estimators of density functions are slower than ordinary parametric estimators, which have root-n consistency. If the appropriate kernel function is used, the kernel estimators of the distribution functions recover the root-n consistency, and the inferences based on kernel distribution estimators have root-n consistency. Further, the kernel-type estimator produces smooth estimation results. The estimators based on the empirical distribution function have discrete distribution, and the normal approximation cannot be improved?that is, the validity of the Edgeworth expansion cannot be proved. If the support of the population density function is bounded, there is a boundary problem, namely the estimator does not have consistency near the boundary. The book also contains a study of the mean squared errors of the estimators and the Edgeworth expansion for quantile estimators.
Author: Rizky Reza Fauzi, Yoshihiko Maesono |
Publisher: Springer |
Publication Date: Jun 01, 2023 |
Number of Pages: NA pages |
Language: English |
Binding: Paperback |
ISBN-10: 9819918618 |
ISBN-13: 9789819918614 |
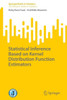
Statistical Inference Based on Kernel Distribution Function Estimators
$60.77
This book presents a study of statistical inferences based on the kernel-type estimators of distribution functions. The inferences involve matters such as quantile estimation, nonparametric tests, and mean residual life expectation, to name just some. Convergence rates for the kernel estimators of density functions are slower than ordinary parametric estimators, which have root-n consistency. If the appropriate kernel function is used, the kernel estimators of the distribution functions recover the root-n consistency, and the inferences based on kernel distribution estimators have root-n consistency. Further, the kernel-type estimator produces smooth estimation results. The estimators based on the empirical distribution function have discrete distribution, and the normal approximation cannot be improved?that is, the validity of the Edgeworth expansion cannot be proved. If the support of the population density function is bounded, there is a boundary problem, namely the estimator does not have consistency near the boundary. The book also contains a study of the mean squared errors of the estimators and the Edgeworth expansion for quantile estimators.
Author: Rizky Reza Fauzi, Yoshihiko Maesono |
Publisher: Springer |
Publication Date: Jun 01, 2023 |
Number of Pages: NA pages |
Language: English |
Binding: Paperback |
ISBN-10: 9819918618 |
ISBN-13: 9789819918614 |